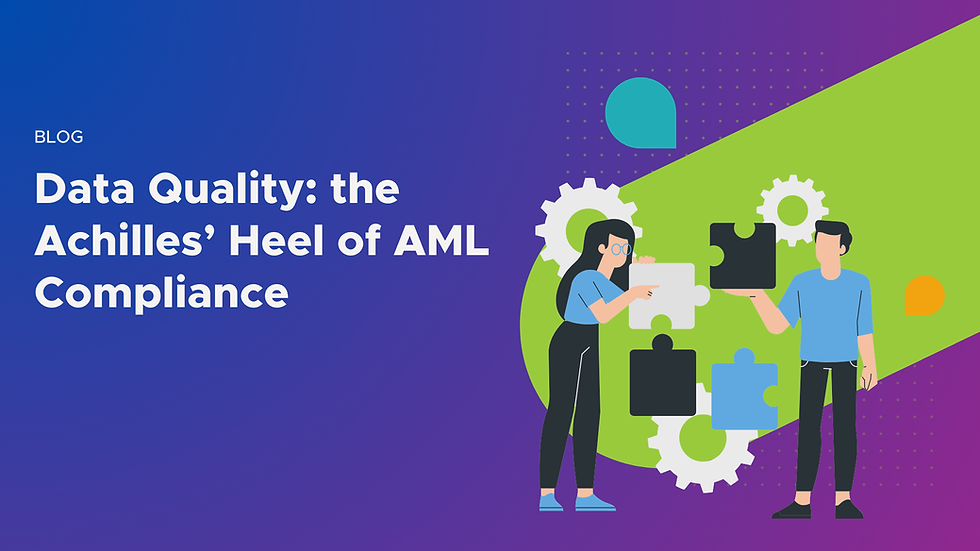
Data quality has been an issue for organizations for decades. Traditional data management approaches often involve separate vendors for data quality, anti-money laundering, and sanctions (AML) compliance, leading to data that may be mastered for business operations but not necessarily optimized for compliance purposes. Today, the stakes are increasing for compliance professionals as they are responsible for the consequences of bad data.
Guidance from regulators and AML industry bodies across the globe, such as the U.S. Office of the Comptroller of the Currency, The New York State Department of Financial Services, and The Wolfsberg Group—just to name a few—expect data quality as an essential component of an adequate AML compliance program. Many regulatory and sanctions enforcement penalties imposed on financial institutions (FIs) and other global companies can frequently be traced back to poor data quality and insufficient data governance measures.
In addition, poor data quality often erodes the effective performance of an organization’s AML program, negatively impacting both risk mitigation and productivity. Individual and entity names hidden in joint accounts, address lines, and around noise words can impair the effectiveness of any matching technology and an organization’s ability to detect risk. What risks are you taking with your compliance data?
The Wolfsberg Group’s strategies for refining AML and CTF
The Wolfsberg Group’s guidance on AML programs provides a comprehensive framework for financial institutions to effectively manage financial crime risks. Its “Effective Monitoring for Suspicious Activity” (MSA) document outlines advanced strategies to refine AML and counter-terrorist financing (CTF) efforts across the sector, moving beyond traditional transaction monitoring towards a comprehensive, risk-based approach. It emphasizes the importance of a wider perspective in identifying and reporting suspicious activities more accurately, which would typically require:
Emphasizing effectiveness over compliance: FIs should pivot towards a risk-based approach (RBA), moving away from rules-based systems focused on compliance. Effectiveness should be measured by the usefulness of the information generated, not just compliance metrics.
Risk-based monitoring: Prioritize monitoring of customers, products, and transactions based on observed risks and typologies. Use data to assess the productivity of suspicious activity monitoring routines, allowing resources to be focused on real, crystallized risks rather than theoretical ones.
Enhanced data analysis: Develop analytical capabilities to perform timely data analysis and investigations aligned with government priorities. Proactively conduct risk identification exercises to spot emerging threats and idiosyncratic risks across the business.
Alignment with national priorities: Understand and align monitoring efforts with national or supranational priorities in combating financial crime. FIs should seek feedback from authorities on the value of their SARs/STRs to refine their monitoring programs.
Data quality and governance: Ensure robust data governance and quality control frameworks. Incorporate not just transactional and customer data but also dynamic information like IP addresses, device IDs, and data from reputable external sources (e.g., UBOs, market data).
Entity resolution and network analysis: Leverage technologies that connect internal and external data, providing a network-based view of customers. This enhances contextual understanding and expedites risk management decisions.
The crucial role of superior data processing and matching
Leveraging advanced data assessment and matching technologies can help improve the quality of data used in your AML processes. A data quality solution should be able to ingest and analyze customer data from various sources, process it to uncover hidden entities and remove noise, and optimize it for effective screening.
Check to see if your data integrity program focuses on the following key components:
Develops and updates comprehensive inventories of systems containing data relevant to key AML compliance systems
Establishes clear roles and responsibilities for the management and oversight of AML data
Identifies high-priority AML use cases and informs the CRM team of projects impacting financial crimes use cases
Documents data dictionaries and data sourcing process maps and desktop procedures related to AML compliance systems
Creates end-to-end data lineage documentation
Remediates data defects within the lines of business and relevant enterprise functions
Maintains procedures and controls to ensure timely and accurate information is provided to the AML compliance Systems
Conducts risk-based data and control testing for completeness, accuracy, and control effectiveness
Provides training to targeted audiences involved in the data supply chain
These components should work in concert to provide your firm with a more precise, efficient, and effective AML process. By addressing data quality as a precursor to ingesting data into an AML solution, risks will be more effectively identified, mitigated, monitored, and reported; efficiencies will be achieved; and regulatory compliance obligations will be met.
An AML solution should also be flexible and configurable, allowing you to tailor it to your organization’s specific needs and risk appetites, and come with robust, integrated model governance features to ensure model transparency (conceptual soundness) and regulatory compliance.
Strengthening AML compliance with data quality, advanced tech
The evolving landscape of regulatory expectations and financial crime risks necessitates a robust approach to data quality in AML programs. Traditional methods of data management are no longer sufficient in addressing the complex challenges posed by poor data quality, which can severely undermine an organization's ability to comply with regulatory requirements and effectively mitigate risk. Hence, compliance teams face three main options:
Accept the risk and widen screening and monitoring rules, which can overwhelm systems with excessive false positives and low-quality results
Address data quality issues at the source ("upstream") through comprehensive data governance projects, which can be costly and require extensive company-wide commitment, making them difficult to justify internally.
Integrate data quality controls directly into compliance tools, enhancing screening and monitoring accuracy without the need for large-scale data overhauls
With the integration of cutting-edge technologies, a focus on data quality, and a commitment to continuous improvement, organizations can build a more resilient and compliant AML framework. By prioritizing data quality and leveraging innovative tools, institutions are better equipped to navigate the complexities of modern financial crime compliance and safeguard their operations against the growing threats of financial crime.
Built on decades of experience in data management and proprietary matching technologies, FinScan provides a data-first, risk-based approach to ensure accuracy and efficiency in identifying and reducing risk, accelerating AML compliance workflows, and optimizing team productivity. Explore the possibilities by booking a discovery call today.